Beta: MediSked Natural Language Processing Technology
The beta NLP tool above is for you to test out writing service notes to examine their sentiment.
DISCLAIMER: This technology is in the early stages of development for Human Services. These results are not intended for clinical decision support, human resource management, or any purpose other than to illustrate and raise the bar on what could be possible in the next decade.
Applications for Machine Learning Processing in Human Services
Sentiment Analysis is an approach to machine learning / natural language processing (NLP) that identifies the emotional tone behind a body of text.
Machine Learning, Natural Language Processing and Sentiment Analysis are in their early stages in 2023, so the purpose of this work is to help advance technologies for home and community-based services – these results do not tell the whole story!
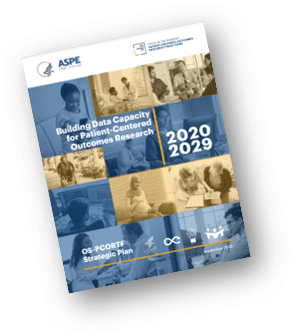
DHHS has Identified Machine Learning as a Goal for Person-Centered Outcomes by 2029
Human Service Providers have Plentiful Unstructured Service Notes / Clinical Notes
- Leverage leading technology solutions to improve data capacity for person-centered outcomes and comparative clinical effectiveness research
- Use AI solutions to enhance accessibility and interoperability of unstructured data to advance person-centered outcomes
Term | Description |
Predictive decision support (Model) | Technology intended to support decision-making based on algorithms that derive relationships from training or example data and then are used to produce an output or outputs. |
Transparency | Sufficient information provided on the model, including input data, validation of performance, and intended use. |
Trustworthiness | Model risks identified, mitigated, managed, and evaluated to provide confidence in the positive impact of using the model, and information about steps taken to govern the model and address negative impacts and/or reduce bias or harm are documented. |
Fair (Unbiased, Equitable) | Model does not exhibit prejudice or favoritism toward an individual or group based on their inherent or acquired characteristics. The impact of using the model is similar across same or different populations or groups. |
Appropriate | Model is well matched to specific contexts and populations to which it is applied. |
Valid | Model has been shown to estimate targeted values accurately and as expected in both internal and external data. |
Effective | Model has demonstrated benefit in real-world conditions. |
Safe | Model is free from any unacceptable risks and for which the probable benefits outweigh any probable risk. |